We bridge four proven tools of international business literature
*
With virtually every product today representing a summation of cross-border value added activities, it becomes vital to break down the complexity in globally dispersed value chains
*
The nature of economic activity undertaken within any industry depends on the availability of related firms in other industries, making it imperative to understand industrial composition
*
Understanding the level of intricacy in global economic systems makes it possible to explain differences in diversification patterns, economic growth and income inequality
*
While this tool has long been used by academics to explain the role of various distances between a pair of countries, we reinvent it by exploring the importance of time over distance
We use each of these cross-complementary dimensions as tools to analyze the SLGL region’s economy in real-time
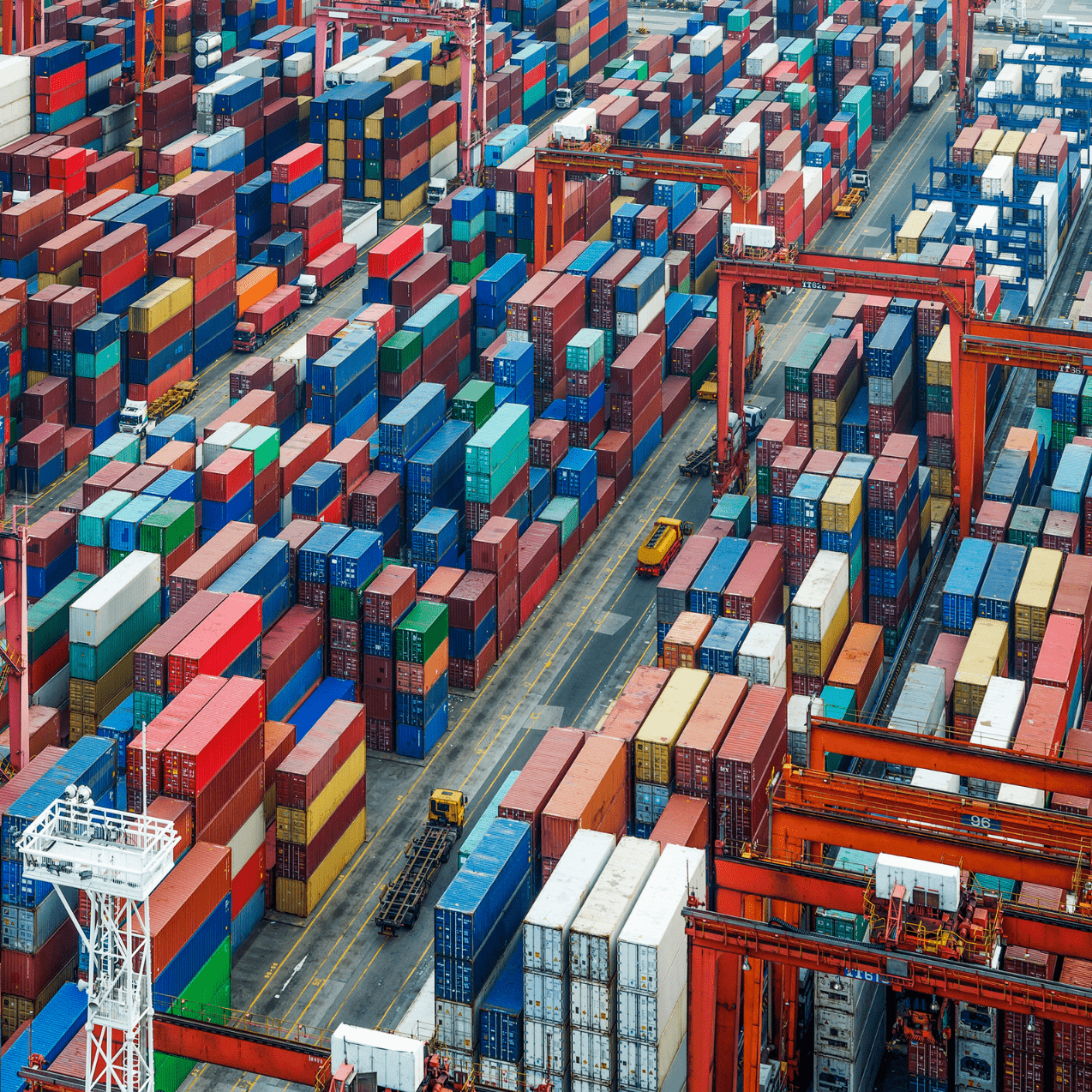
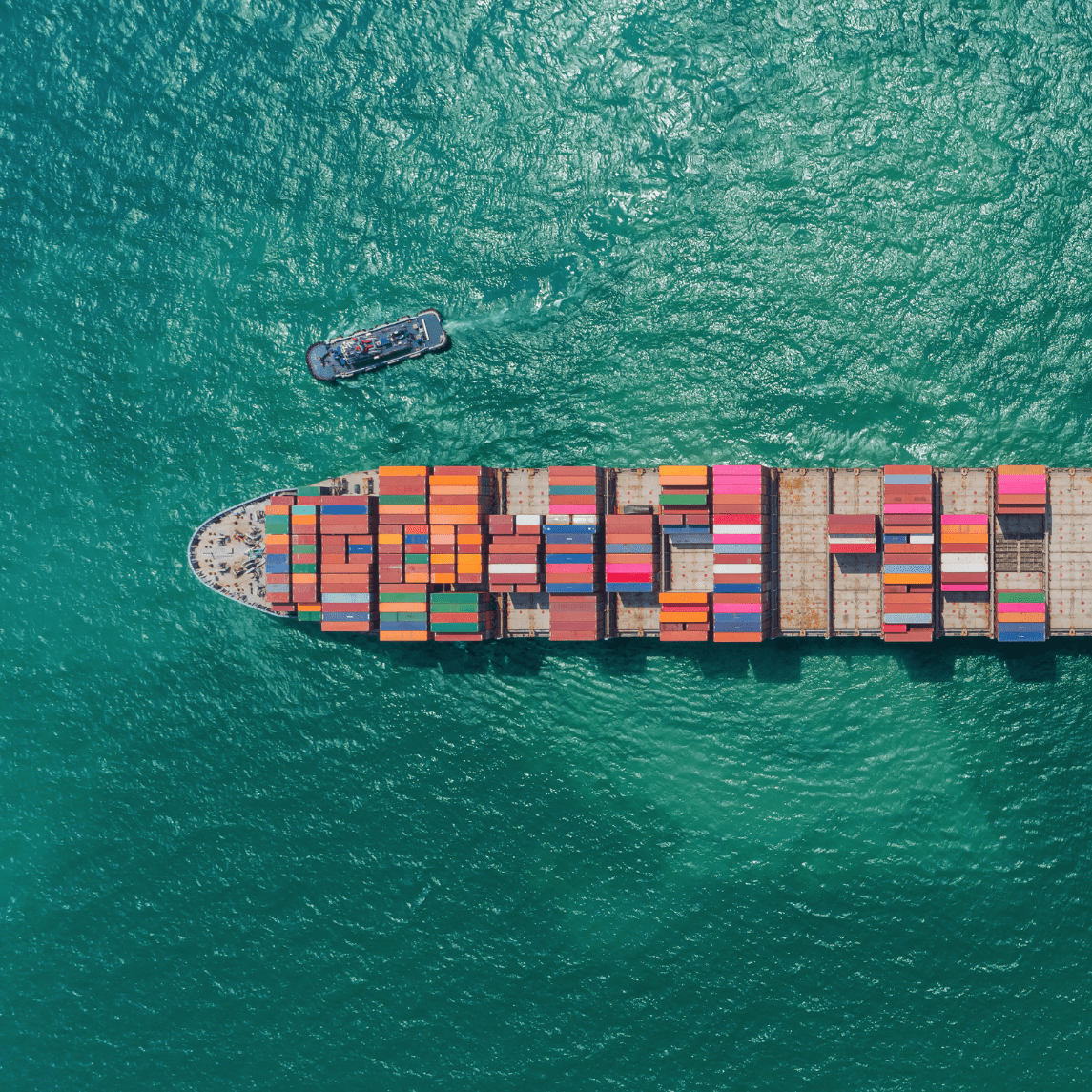
*
Global Value Chains
The decentralized cross-border fragmentation of a company’s value chain is a powerful source of efficiency and competitiveness. Companies have structured and outsourced their internal processes around these Global Value Chains (GVCs) to pursue comparative advantages of locations, despite of the increased complexity in managing operations and susceptibility to shocks in the global economy. GVCs increasingly account for the growth in international trade, global GDP and employment. This makes it an interesting dimension for the study of a region’s economy.
The GVC framework allows to study the organization of global industries through the analysis of the structure and the dynamicsof complex interactions between actors involved in a given supply chain. The measurement of value in a supply chain lies in the sequential value added by each actor in the form of inputs, from a product’s conception to its consumption. Mapping this input-output structure shows not only the flow of tangible and intangible goods and services but also shows the true value of an economy’s output.
Of late, GVCs are increasingly becoming regionalized due to a host of factors that include the shifting deadlocks in geopolitics, the undergoing recovery from the COVID-19 pandemic and the rapidly advancing consequences of climate change. Policy and business strategies are failing to produce their intended effects as they have been designed without accounting for the inherent complexities in the underlying linkages of GVCs. To counter the uncertainty produced by such distortions, a tool that helps visualize value chains becomes imperative.
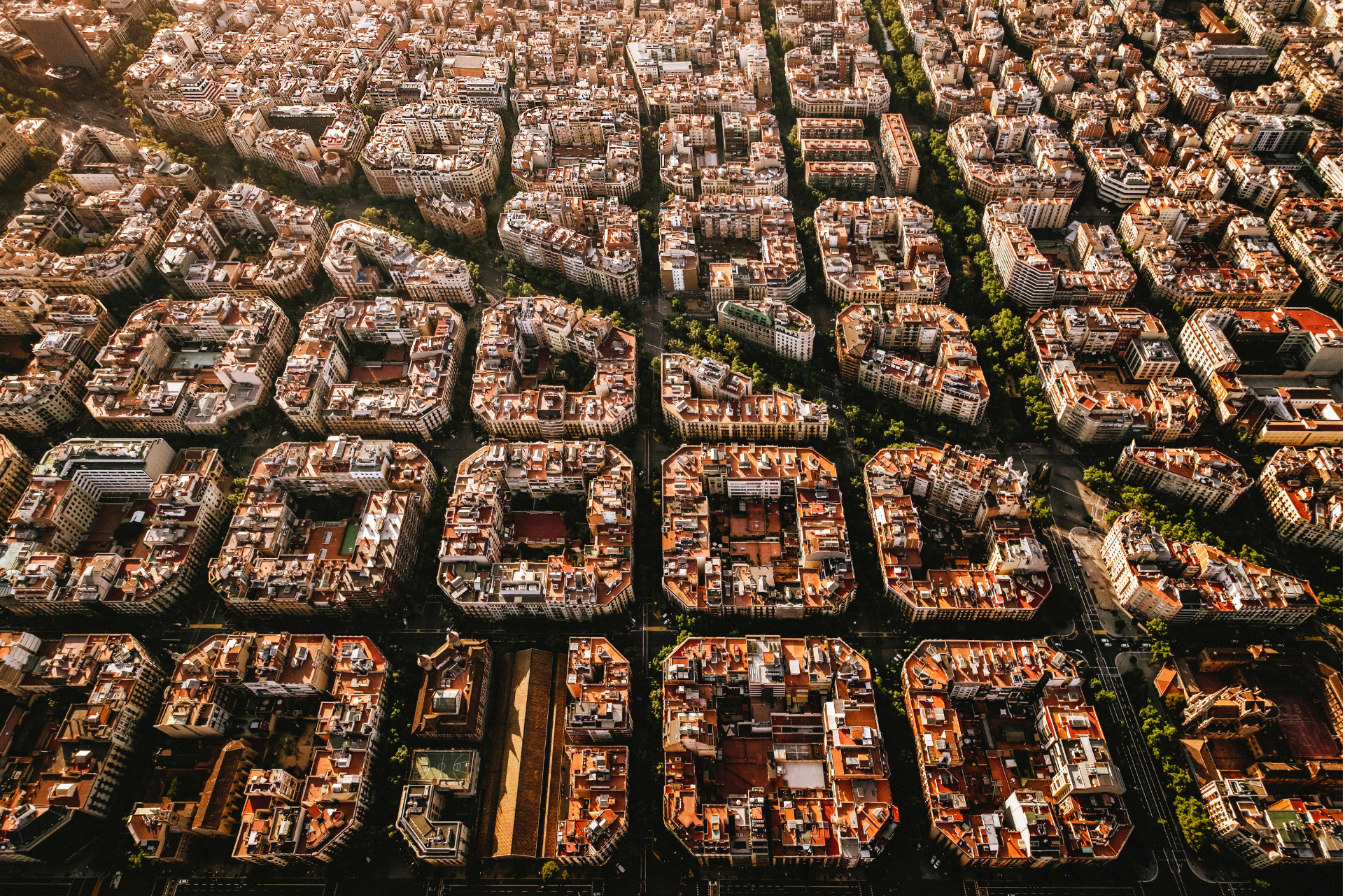
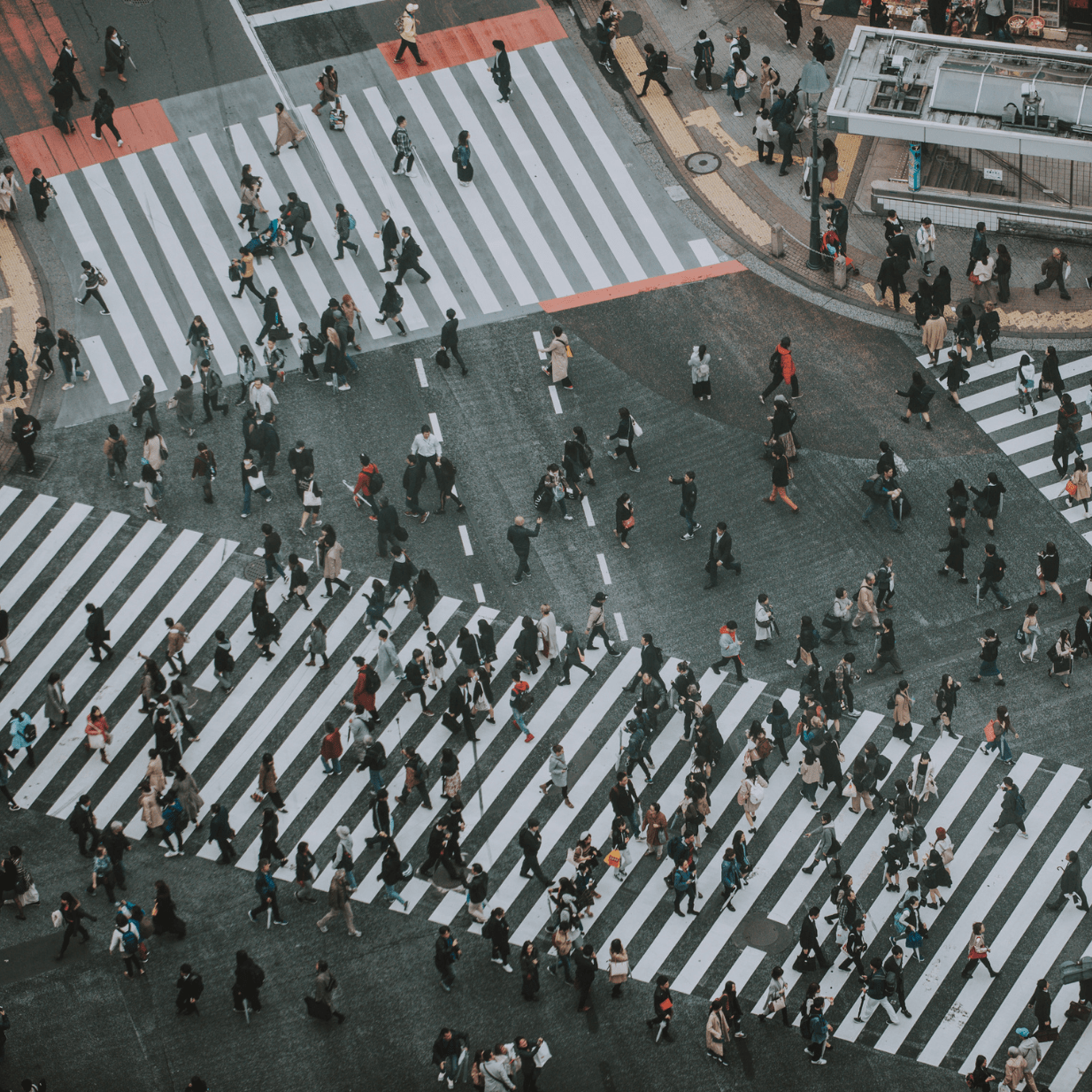
*
Economic Clusters
Clusters are a geographic concentration of companies, specialized suppliers, service providers, firms in related industries and associated institutions that are linked together through cooperation and competition within a particular industry. These linkages ease the movement of knowledge, technology and talent, leading to spillovers that form the foundations for local collaboration. In fact, an industry’s ability to produce complex and high-value products becomes contingent on the presence of strong interrelated clusters.
Clusters offer a key opportunity to peruse the relationship between competitiveness, productivity and prosperity. Globalization has resulted in an uneven distribution of economic activity and consequently economic performance and competitiveness across regions. Policymakers have increasingly turned their focus towards clusters as a tool to manage a rapidly changing economic landscape on a sub-national level. Therefore, any effort of crafting cluster policy requires a comprehensive understanding of economic activity within a region.
Our use of real-time data on firms with machine learning models to group them offers an alternative perspective towards the measurement of clusters. This helps account for a defining characteristic of clusters that they are not limited to the arbitrary geographical boundaries of a region, state or county and industrial classification systems. This inductive research approach lies in stark contrast to the current standards of measuring clusters that are bound to definitions that conform to industrial classification systems and arbitrary geographic boundaries.
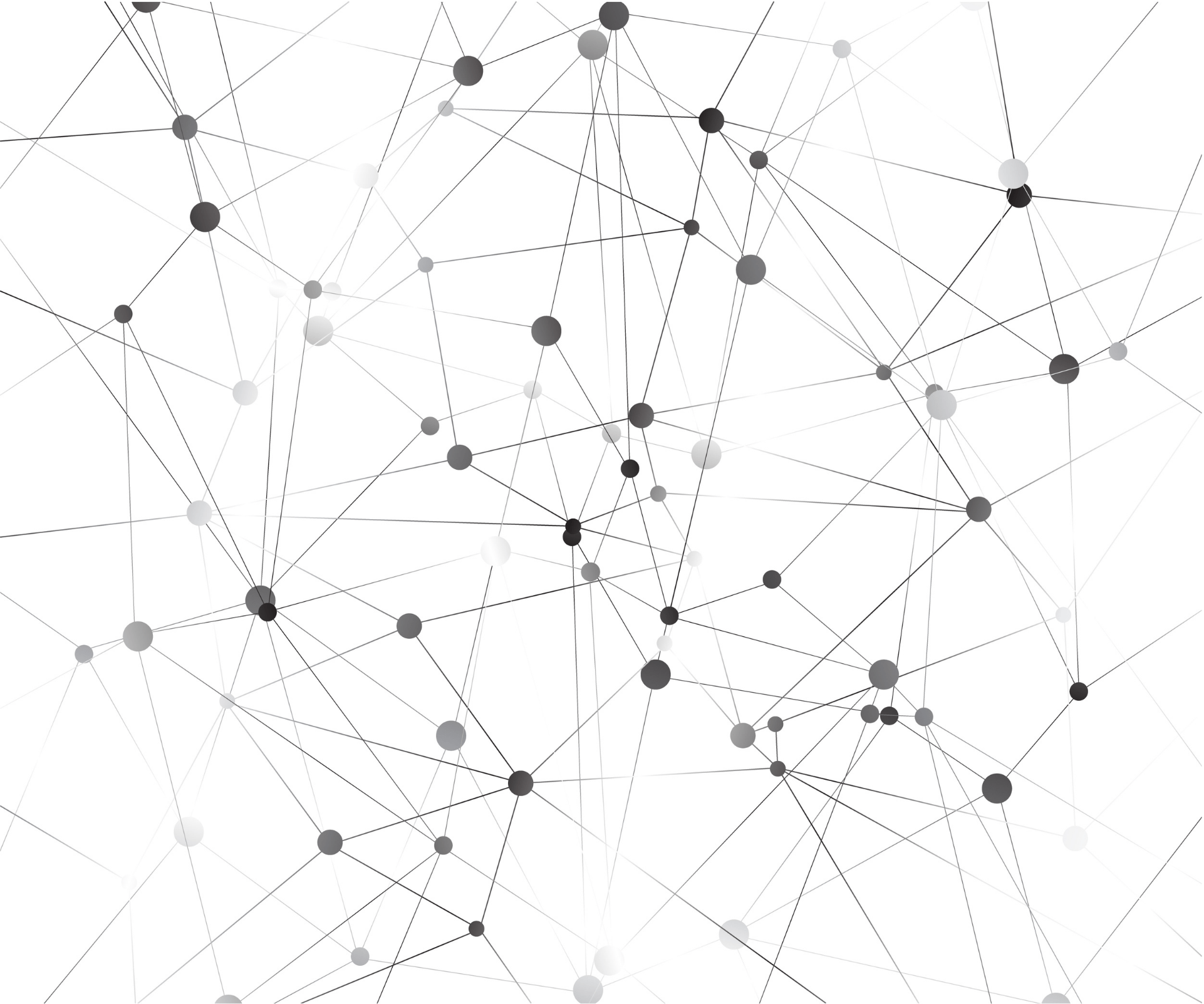
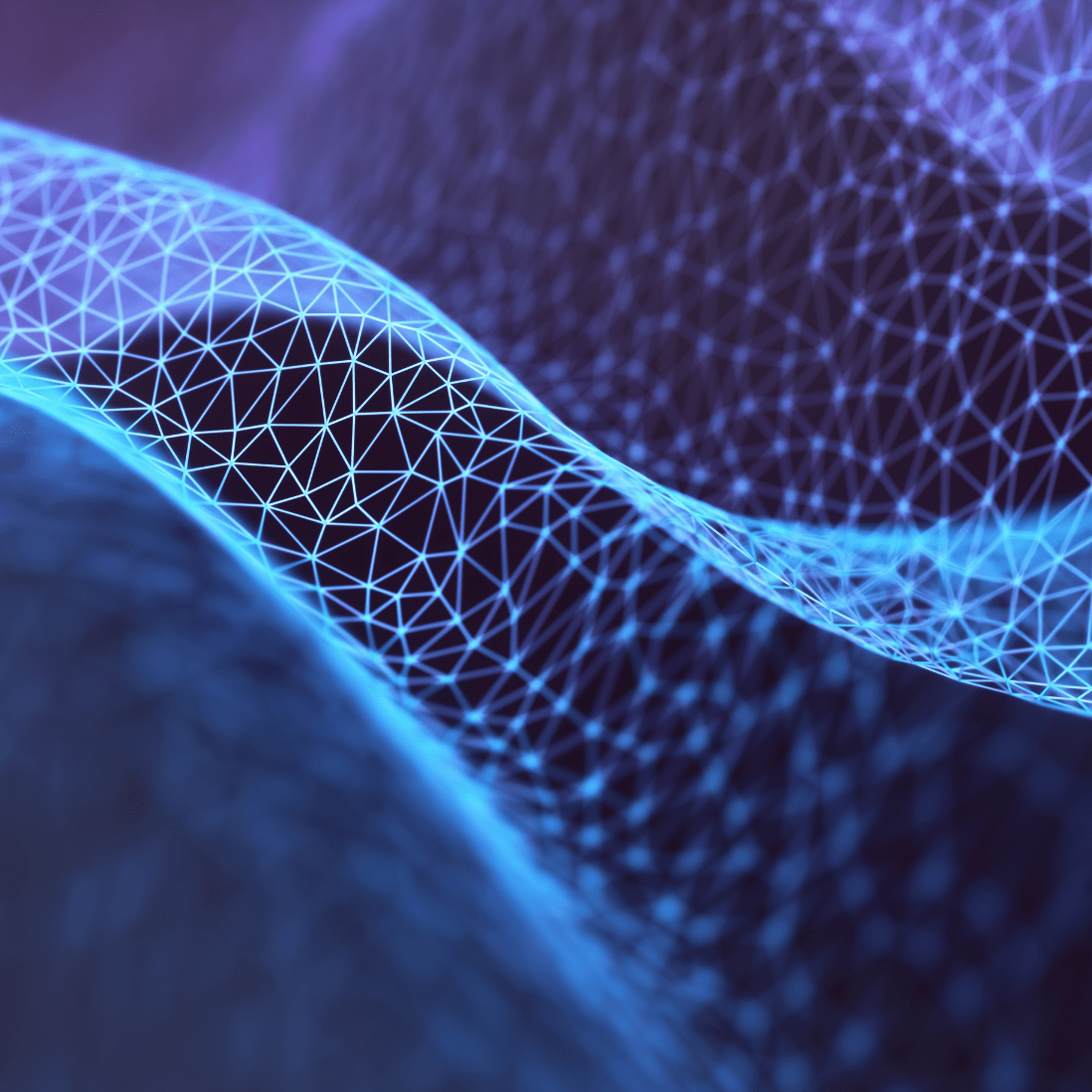
*
Economic Complexity
The modern economy of a country is a myriad of complex internationally interconnected economic systems. These systems have variance in the nature of its economic activity that can be traced down to the difficulty in transferring and combining different pieces of explicit knowledge that people hold. Highly complex products require coordination between hundreds of people leveraging their expertise to create individual components of the product.
Economic complexity is a measure of the level of intricacy of such networks of interactions needed for a region to mobilize its productive knowledge. A region’s economic complexity is expressed in the composition of its productive output and reveals the underlying network of interactions required to combine disparate pieces of knowledge. Economic complexity is studied through the lenses of product diversity and networks of relatedness.
Economic complexity is a powerful quantitative tool that heavily relies on big data and machine learning models to explain differences in diversification patterns, economic growth and income inequality. Metrics of complexity provide key estimates about the overall potential of an economy, by measuring the combined presence of economic factors by being agnostic about what those factors may be. It allows to introduce relevant heterogeneity between industries and products by summarizing the vectors that best explain the geography of thousands of economic activities.
We contribute to the literature on economic complexity by adding transportation to the block of analysis. This is an important consideration to make as geography plays an important role in the diffusion of knowledge. It is well understood that advances in transportation promote geographic spillovers by effectively warping distance. Also, by studying the input-output structure within the SLGL region, goods that are not exported to other countries also come under our purview.

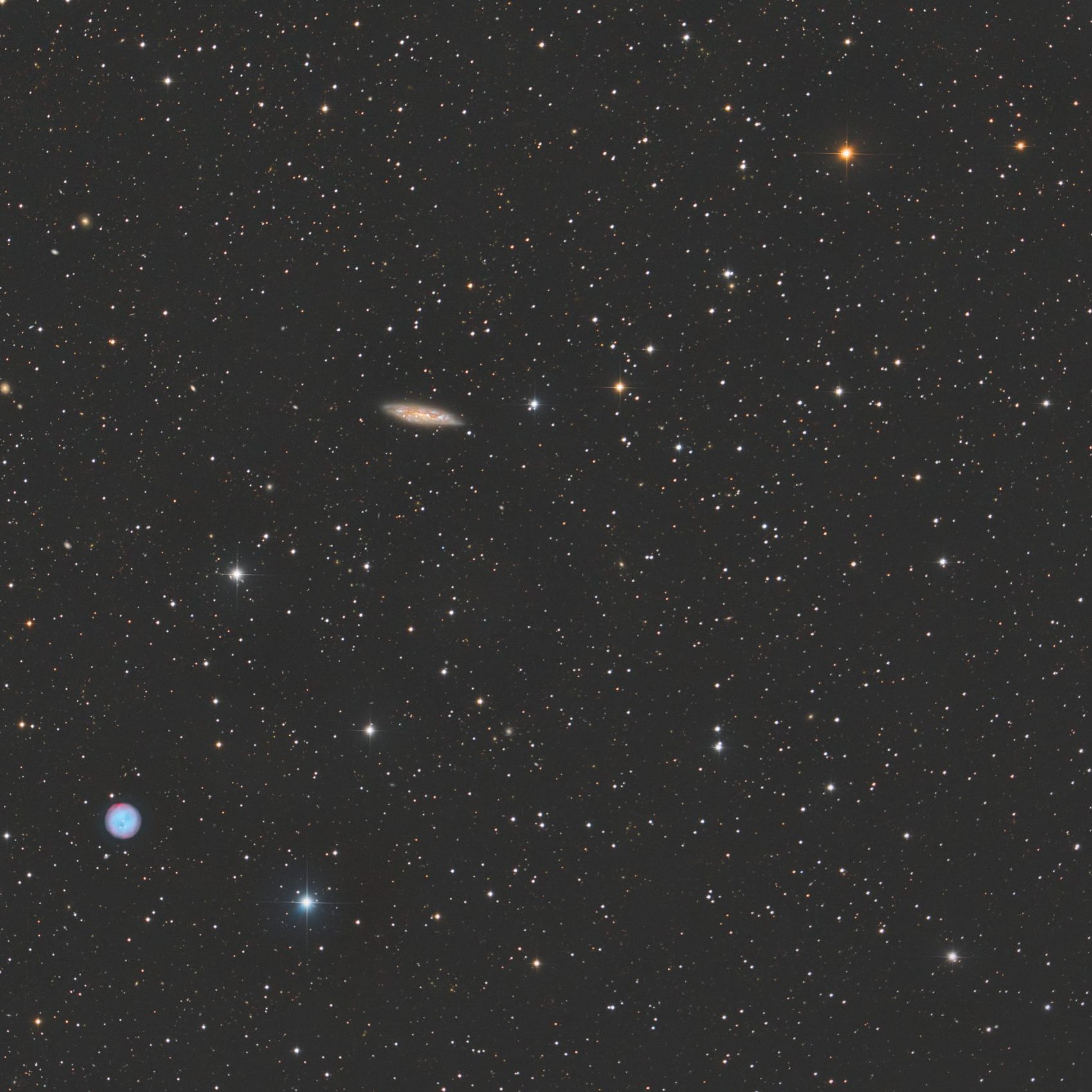
*
Gravity Model
The gravity model has long been used in international business literature to explain the effect of various distances between a pair of countries. The traditional gravity model posits that bilateral trade between two countries is proportional to their economic size (in terms of GDP) and inversely proportional to the geographic distance between them. However, subsequent variations of the model have looked at distance beyond its geographic denotation. The model can be augmented with proxies for geographic distance, leading to a more sophisticated and complex understanding of distance.
Between the two systems of trade and transportation, geographic distance is a common variable that plays a crucial role. Within this context, distance can mean transportation modes, intermodal transportation capacities, supply chain cybersecurity, supply chain decarbonization and so on. The proliferation of digital data has allowed access to information such as the actual distances between two ports. Data science can be used to accurately determine the actual distance travelled by a container ship when transporting goods between two countries.
This optimization brings to light the role of time in trade and transportation as with a decrease in transportation time, trade can be expected to go up. We explore the importance of time over distance by reinventing the gravity model with our real-time geospatial data. With machine learning, estimations are significantly higher in accuracy than with vanilla econometrics models. Reconsidering the distance variable in the gravity model with time can help redefine the role of distance.